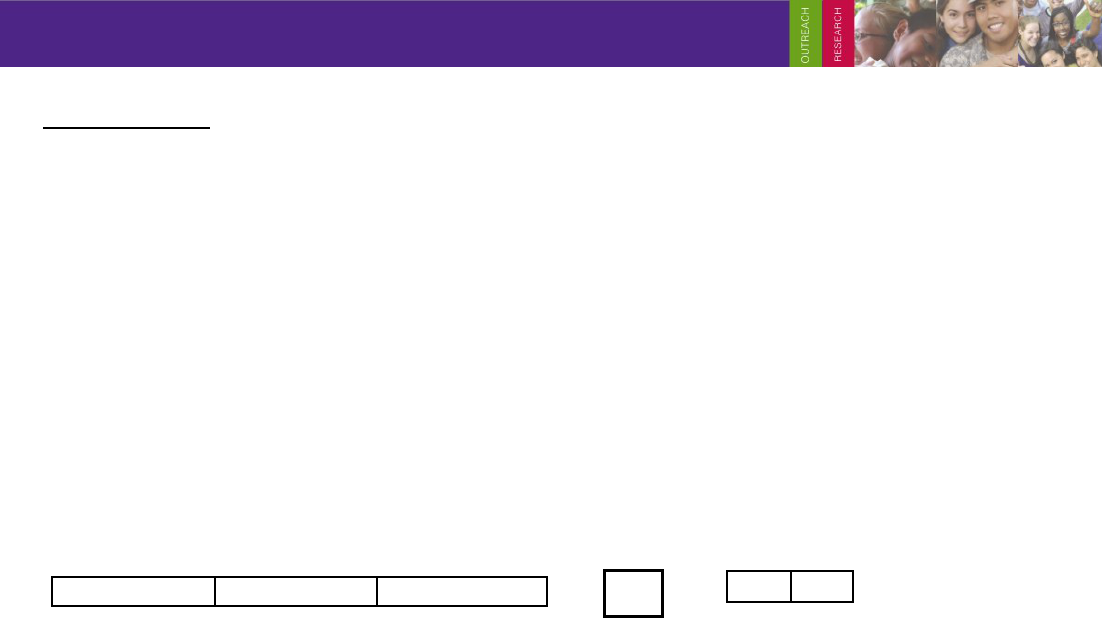
U N D E R S T A N D I N G A N O V A
Factorial ANOVA
Use
Unlike a One-Way ANOVA, a Factorial ANOVA is used when there is more than one independent
variable. In the previous example there was only one independent variable with three levels
(Intervenon A, Intervenon B, control group). Now suppose that a researcher also wanted to know if
there were addional group dierences between boys and girls in the youth program. When this second
independent variable is added to the analysis, a Factorial ANOVA must be used.
Example
Factorial ANOVA’s typically use a mathemacal notaon to indicate the kind of Factorial ANOVA being
conducted. In the present example 3 x 2 Factorial ANOVA is being conducted. Below is an example of
how to break down this notaon:
In this example there are two independent variables, one with three levels (Intervenon A, Intervenon
B, control group) and one with two levels (boy, girl). Thus, the analysis would be a 3 x 2 Factorial
ANOVA.
The results of a Factorial ANOVA consist of several parts. First are called main eects. Main eects tell
you if there is a dierence between groups for each of the independent variables. For example, a main
eect of intervenon type (intervenon A, intervenon B, control group) would indicate that there is a
signicant dierence between these three groups. That is, somewhere among these three means, at
least two of them are signicantly dierent from one another. Like the One-Way ANOVA, a Factorial
ANOVA does not provide informaon on where these dierences are and addional analyses are
required, these addional analyses are discussed below (Post-Hoc Analyses and Planned Contrasts)
A main eect of gender would indicate that there is a dierence between the means of boys and girls.
In addion to the main eects, Factorial ANOVA also oen provides informaon about interacon
eects as well. Interacon eects provide informaon about whether an observed group dierence in
one independent variable varies as a funcon of another independent variable.
An example will clarify:
Suppose that a researcher found a main eect for intervenon type. This tells us that there is a signi-
cant dierence in instrucon quality between these three groups. If there were a signicant interacon
between intervenon type and gender, this would mean that the dierences found between interven-
on types were only there for one gender and not the other. Below is an example of such an interac-
on.
4
Intervenon A Intervenon B Control Group
By
Boy Girl
3
x
2